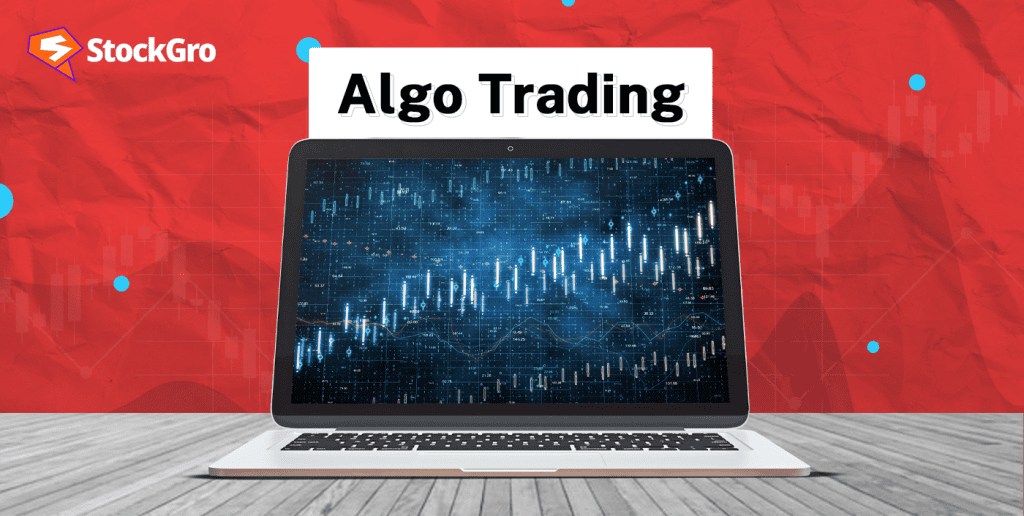
Introduction
By 2032, the global algorithmic trading market between 2024 and 2032, growing 15.9% to $65.2 billion. India’s algorithmic trading rate rose from 10% in 2011 to 50% in 2019.
AI and machine learning allow traders to assess massive data sets in real time, transforming algorithmic trading. As markets get more complicated, algorithmic trading precision and success become more important.
In algo trading, computer algorithms conduct deals depending on price, time, or volume. Algorithmic trading is necessary in financial markets to expedite execution, reduce human error, and automate intricate operations.
Let us delve further into understanding algorithmic trading.
Understanding algorithmic trading
What is algo trading?
Algo trading processes trades based on price, volume, or time. Trading by machine is faster and more precise. Automation eliminates emotional biases and facilitates best-case bets in volatile markets.
The main elements of algorithmic trading are as follows:
- Trading algorithms: Predetermined market-responsive transaction instructions.
- Platforms: Software systems that execute and optimise algorithms.
- Execution methods: These systems guarantee that transactions are executed in real time without any delay.
Algorithmic trading provides distinct advantages over conventional methods:
- Speed: Algorithms are capable of executing transactions at a significantly faster pace than humans.
- Accuracy: Trades are executed with precision due to pre-programmed rules.
- Emotional bias removal: Automated trading eliminates emotional decisions, such as dread or greed.
- 24/7 monitoring: Algorithms are capable of monitoring and responding to market conditions at all hours of the day.
Recently, institutional and retail traders have turned to algorithmic trading for its capacity to manage complicated market circumstances and process massive volumes of data. Cloud computing and AI let traders execute deals quickly and precisely, driving this trend.
These trends advise relying more on automated systems to capitalise on transient market possibilities, decrease transaction costs, and manage hazards in fast-moving situations. Institutional and retail investors use algorithmic trading to enhance performance and make data-driven decisions as technology advances.
Learn more about : Automate your unique trading strategies with the help of algo trading.
Key factors driving algo trading in 2024
The primary factors influencing algorithmic trading in 2024 are:
–Technological innovations: The performance of algorithms is being considered enhanced by advancements in AI, machine learning and big data analytics. AI allows algorithms to learn from data, adapt to market conditions, and predict trends with greater precision. Complex trading techniques require machine learning algorithms to automate planning and manage vast datasets.
– Global uncertainty: Global economic and geopolitical instability in 2024 increases market volatility. Due to its speed, algo trading capitalises on price swings and reduces human mistakes in volatile markets.
–Regulatory environment: The landscape for algo traders is being influenced by the introduction of evolving regulations by global financial authorities. Stricter transparency and risk management rules globally are influencing algorithm operation, resulting in more secure and compliant trading tactics.
-Market liquidity: Algorithmic trading speeds up large transactions, reducing the impact of large orders on price changes. Institutional and retail investors benefit from this liquidity because it ensures market stability, especially during high reading volumes.
Top algo trading strategies for 2024
- Trend following algorithms
Among top algo trading strategies, trend-following algorithms profit on long-term market trends like price increases and decreases. Technical indicators including momentum indicators, relative strength indexes, and moving averages help these methods spot trends and set entry or exit points.
–Time frame and markets: Trend-following methods work best in liquid markets like equities, commodities, and FX, where trends last longer. Timeframes range from intraday to multi-week or multi-month depending on trend intensity and longevity.
–Risk management tips: Stop-loss orders and trailing stops lessen trend reversal risks. Diversifying assets across markets or industries reduces market risk.
Arbitrage techniques profit on market or asset price differences. If two exchanges price an asset differently, speculators may buy it on one and sell it on the other for profit. These methods sometimes use high-frequency trading to perform transitions before the price gap dissipates.
To find arbitrage possibilities, traders focus on currencies, commodities, and derivatives with pricing inefficiencies. Monitor cross-border asset pricing, corporate activity, and interest rate differentials for profitable arbitrage opportunities. For example, a soybean speculator who buys at ₹500 a bag and shorts the future contract at ₹600 makes ₹50 per unit after deducting ₹50 for storage fees. The Indian commodities market often uses this strategy to capitalise on the price difference between current and future markets.
In India, cash-and-carry commodity arbitrage is a common strategy.
Understand more about: How does arbitrage trading work?
Mean reversion techniques assume asset values will return to historical averages. This technique involves selling assets when they are overvalued and buying them when they are undervalued, especially when prices diverge greatly from this average, expecting the price to return to its mean.
In order to develop effective mean reversion strategies, traders implement statistical measures to identify potential buying and selling points. Standard deviations are employed by traders as a critical tool for determining the degree to which an asset’s current price has deviated from its historical average.
Moving averages are also evidently essential in the context of evaluating price deviations from longer-term trends, which is why traders employ short-term averages.
A potential reversion is indicated when a stock’s short-term moving average significantly diverges from its long-term average, which provides traders with guidance on when to enter or exit positions. Statistical measures such as these provide a structured method for leveraging price fluctuations in mean reversion strategies.
- Market-making algorithms
Market-making algorithms persist in providing both the bid and offer prices for specific assets in order to enhance liquidity in the market. This algorithm ensures that the asset inventory is balanced while simultaneously generating revenue from the spread, which is the discrepancy between the bid and ask prices.
–Asset selection: Concentrate on assets that are highly liquid, such as blue-chip stocks or significant cryptocurrencies, where volume is high and spreads are tight.
–Risk management: To prevent excessive exposure, particularly during volatile market fluctuations, implement automated stop-loss mechanisms and position limits.
–Spread dynamics: Optimise profits by dynamically adjusting spreads to market conditions, increasing during periods of volatility and tightening during periods of stability.
- Momentum-based strategies
Momentum trading capitalises on sustained upward or downward movement in assets by exploiting price trends. Traders initiate a position when momentum is accumulating and abandon it when it begins to diminish.
-Trade entry and exit: It is essential to enter a trend at the beginning and exit before it reverses. The identification of the appropriate action points is facilitated by the use of indicators such as the moving average or RSI.
–High-speed data management: In order to guarantee rapid execution, momentum trades are exceedingly susceptible to rapid market fluctuations. Consequently, traders must implement real-time data inputs.
In 2023, Bitcoin was a notable success due to the fact that momentum traders capitalised on the increase in the wake of institutional adoption announcements. These traders made consistent profits by entering the market during quick price surges and quitting before major corrections.
Statistical arbitrage exploits price inefficiencies between interlinked assets using mathematical models. These strategies are frequently implemented on pairings of securities, employing regression models and mean reversion to anticipate price discrepancies.
–Quantitative analysis: Traders employ statistical models, such as regression analysis or cointegration tests, to identify mispriced pairs.
–Pairs trading: This frequently used method trades two highly correlated assets simultaneously. Purchase the inexpensive item and sell the inflated one.
–Risk management: Set stop-loss orders and consistently update models with new data to prevent potential losses in the event that the predicted reversion does not occur.
Risks and challenges in algo trading
Market risks
Algo trading is especially vulnerable to market risks, such as flash crashes, which cause significant price fluctuations within a brief period by causing precipitous market movements. This price distortion is the consequence of a liquidity trap, which is initiated when an algorithm places substantial orders in illiquid markets.
Slippage, which is the discrepancy between the anticipated and actual executive price, is also a factor that affects algorithmic transactions, particularly in volatile markets.
Technical risks
Algorithmic trading is sensitive to system malfunctions, algorithmic flaws, and connectivity issues as a result of its significant reliance on technology. The potential consequences of these technical glitches include considerable losses, missed opportunities, or incorrect positions, as well as potentially failed transactions.
Regulatory concerns
In 2024, the challenge of adhering to the changing global regulations is becoming increasingly prevalent. Regulatory authorities necessitate transparency and risk controls to prevent market manipulation, necessitating that firms guarantee that their algorithms comply with stringent regulatory standards.
Risk management
In an effort to evaluate market conditions and mitigate losses, volatility measures and stop loss orders are implemented in algorithmic trading. The financial repercussions of unforeseen market events can be mitigated by consistently updating algorithms and implementing rigorous risk protocols.
Understand more about: Key risks in investing in the stock market
Ethical considerations in algorithmic trading
The increased use of machine learning and AI has created new ethical issues in algorithmic trading in India. As algo trading accounts for 50-60% of market volume raises transparency, fairness, and manipulation concerns.
SEBI has instituted rigorous regulations to supervise the application and utilisation of algorithms, thereby preventing these automated systems from unduly exploiting market inefficiencies or destabilising markets.
The market integrity and protection of small investors are being closely monitored in order to address issues like order flooding, dysfunctional algorithms, and excessive speed in high-frequency trading. In order to prevent misuse, the regulator mandates that algorithms endure conformance tests, thereby guaranteeing that ethical practices prevail as technology advances.
Future trends in algo trading
Trading strategies are poised to undergo a revolution as AI-driven algorithms improve real-time decision making and predictive analytics. Machine learning models will enable algorithms to dynamically adjust to changing market conditions, thereby increasing the efficiency and accuracy of transactions.
For instance, AI is anticipated to enhance strategies by analysing immense quantities of financial data in real time, a development that has already started to revolutionise niche trading markets.
Quantum algorithms can solve complex financial equations at unprecedented speeds, revolutionising algorithmic trading. The ability of quantum computers to process large data sets concurrently allows for more advanced market simulations and optimisations.
The emergence of fully automated markets raises ethical issues about the potential loss of human supervision, market manipulation, and fairness. Given the rising deployment of AI and automation in trading, regulatory monitoring and accountability in algorithmic decision-making must be addressed. Consequently, transparency and responsibility are essential.​
Conclusion
In the future, traders will require algorithmic trading strategies such as market-making, arbitrage, trend-following, and mean reversion to capitalise on market fluctuations. All strategies have the capacity to capitalise on price inefficiencies and long-term trends. AI, machine learning, and high-speed data helps traders execute trades more precisely.
Traders must automate risk management, stay abreast of emerging technologies and laws, and create market-focused algorithms to succeed. In order to optimise rewards and mitigate risk, algorithmic trading emphasises speed and data-driven, well-informed decisions in volatile markets.